Prospects of Artificial Intelligence Applications in Agriculture
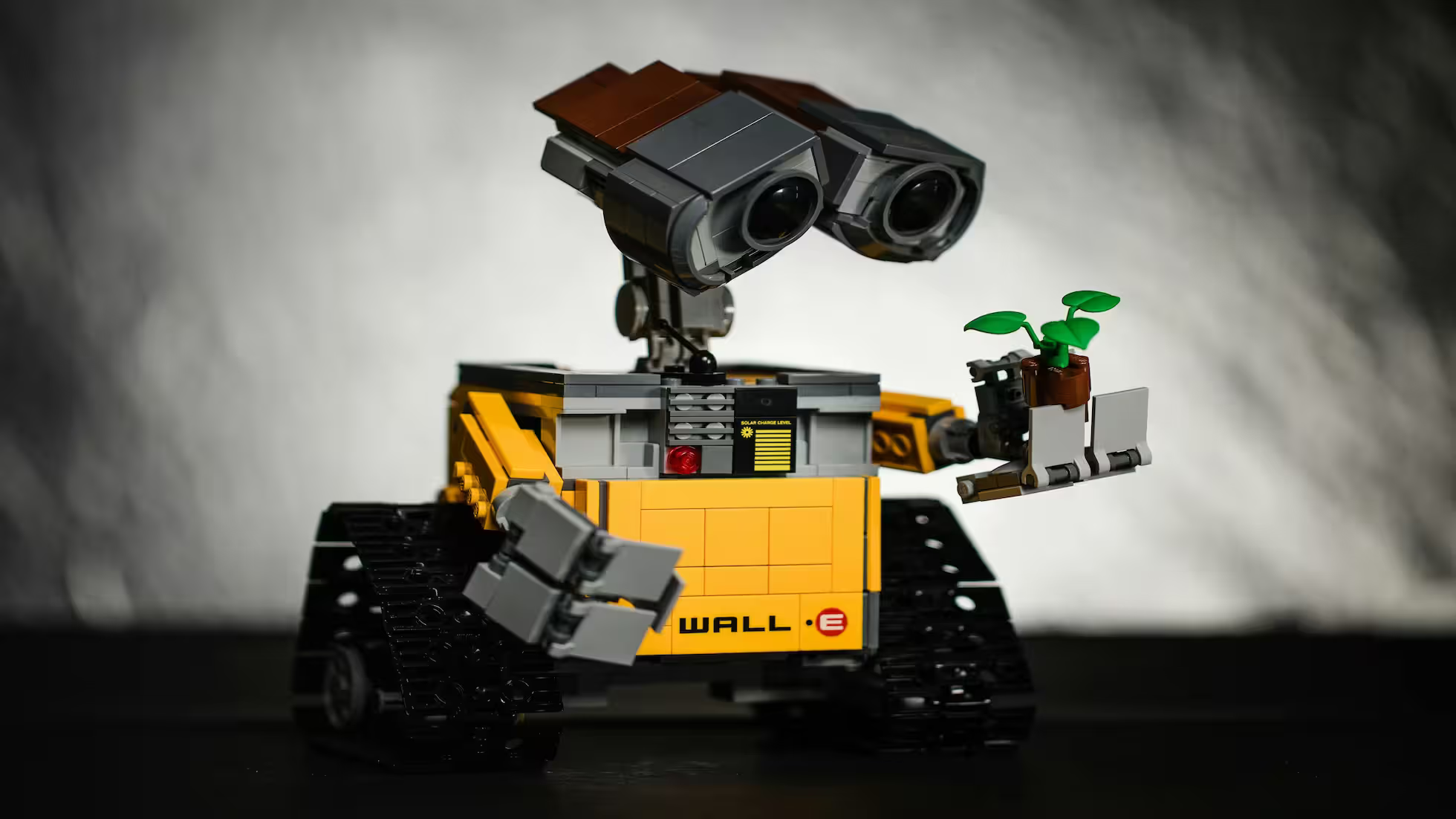
1. Big Data Intelligence
Big data intelligence represents the deep integration of data mining and artificial intelligence technologies. It evolves from shallow computation to deep neural reasoning, from purely data-driven models to a combination of data-driven and knowledge-guided learning, and from task-specific intelligence to more generalized artificial intelligence. Applying big data intelligence technology addresses issues of uncertainty and ambiguity in spatial data and explores solutions to complex, multidimensional, nonlinear problems, paving the way for the implementation of smart agriculture. By uncovering hidden patterns in attribute and spatial data related to agricultural production, big data intelligence facilitates the formulation of precise agricultural strategies and accurate predictions. This enables sustainable, efficient, and coordinated agricultural production, forming the foundation of theoretical research in smart agriculture.
2. Swarm Intelligence
In today’s interconnected world, enabled by the internet and mobile communications, human collectives, big data, and the Internet of Things (IoT) are deeply interwoven. Swarm intelligence has significantly transformed the informational environment of artificial intelligence, presenting new opportunities for agricultural innovation. It provides a novel model—digital agriculture—that leverages collective wisdom to address agricultural challenges. Applications of swarm intelligence include online and offline agricultural product trading, real-time monitoring of agricultural product safety, and logistics review and management.
3. Cross-Media Intelligence
With the progress of civilization and technological advancements, information dissemination has evolved from single-media forms such as text, images, audio, and video to increasingly integrated multimedia formats. This shift highlights the importance of cross-media analysis and reasoning, which has become a key area of research and application. Agricultural machine vision systems are a core technology for achieving cross-media analysis and reasoning. By applying machine vision to various media platforms, images of target crops are captured and processed through specialized image processing systems to obtain morphological data, which is then converted into digital signals. These signals are analyzed to extract features, enabling crop pest and disease diagnosis to aid decision-making. Cross-media intelligence significantly enhances the capability to analyze and process static and dynamic images in agriculture, fostering the application of hyperspectral imagery in the field.
4. Hybrid-Augmented Intelligence and Autonomous Intelligence
Given the uncertainty, vulnerability, and openness of many challenges faced by humanity, no machine, regardless of its level of intelligence, can entirely replace humans. This necessitates the integration of human input or cognitive models into AI systems, creating hybrid-augmented intelligence. This approach represents an essential growth model for machine autonomous intelligence. A prominent example in agriculture is the application of drone technology. Drones combined with imaging spectrometers enable precise, real-time monitoring of spectral information over large agricultural areas, efficiently capturing high-resolution data. Researchers can use this data to establish statistical models for specific goals, developing innovative low-altitude hyperspectral remote sensing platforms based on drones. This advances the application of hybrid-augmented intelligence and autonomous intelligence in agriculture. As this technology evolves, related advancements such as autonomous vehicles, service robots, space robots, ocean robots, unmanned workshops, and intelligent factories are expected to find broader applications in agriculture.